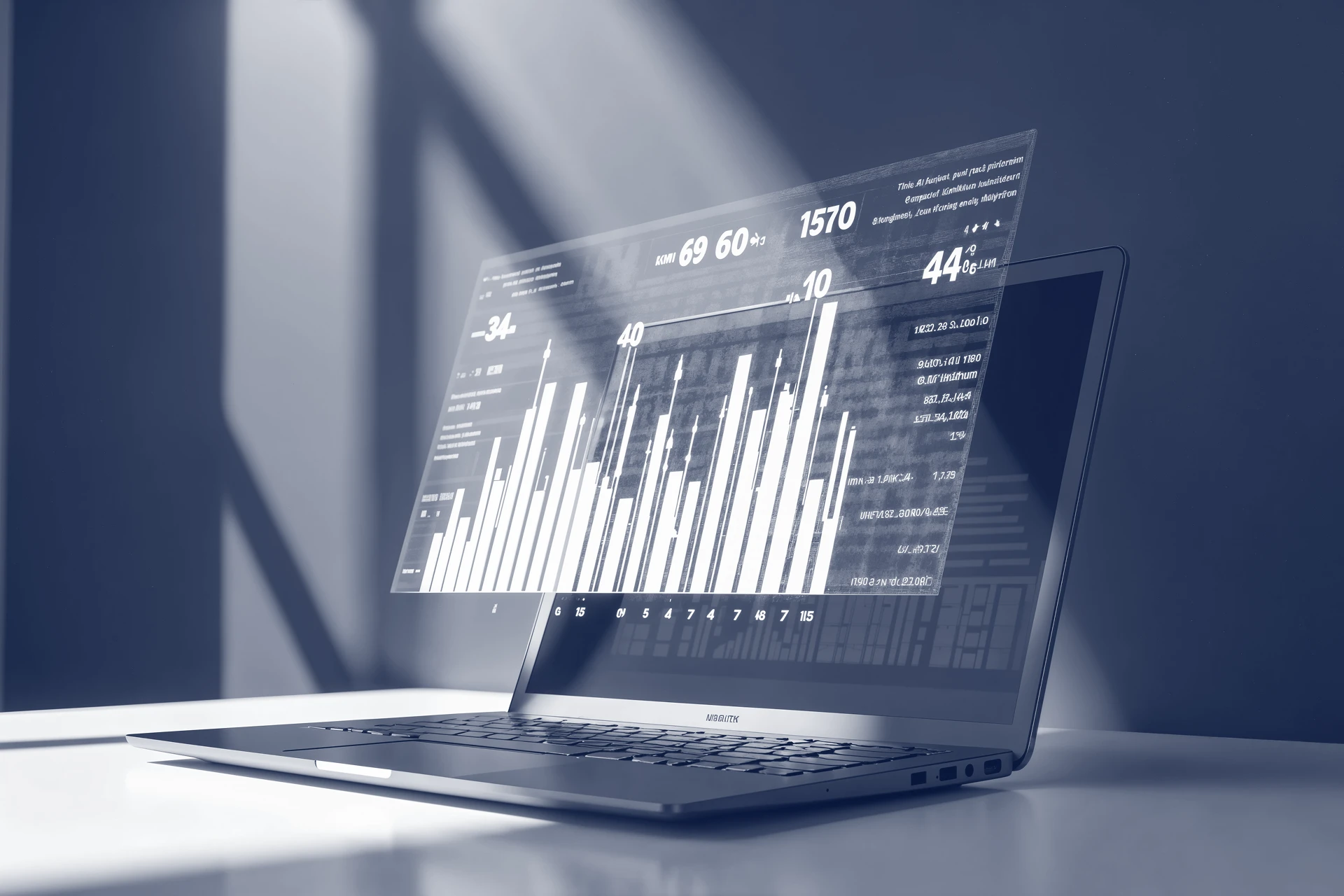
Dynamic AI Pricing: A Smarter Strategy for Unpredictable Workloads
Software and Internet companies are on the brink of a massive revenue shift: according to recent projections, generative AI alone is expected to drive more than a 20-fold increase in revenue over the next three years, with positive ROI expected as early as 2025.
But there’s a catch. As AI adoption accelerates, one thing is becoming clear: most pricing models haven’t kept up. Traditional SaaS tools follow relatively predictable usage patterns. AI workloads, on the other hand, are volatile, bursty, and constantly evolving.
Training a new model, running inference at scale, or spinning up experimental projects can cause sudden spikes in demand. Within a single organization, AI usage can vary wildly between teams and use cases. Yet many vendors still rely on rigid, static pricing tiers built for a more stable past.
The result is that customers get hit with surprise overage fees or end up paying for capacity they don’t actually use. It’s not just inefficient, it’s a trust breaker.
However, change is coming. According to McKinsey, 85% of software companies plan to adjust their pricing strategies in the next two years. More and more companies are moving towards dynamic AI pricing: a more adaptive, transparent, and scalable approach to monetization. Instead of locking users into predefined tiers, dynamic pricing adjusts based on real-time usage, aligning cost with value delivered. It’s not just a billing tweak, it’s a smarter, more strategic approach to pricing that reflects how AI is actually used.
In this blog, we’ll explore what makes dynamic AI pricing different, how to implement it effectively, and why it’s quickly becoming essential for both vendors and customers.
Rethinking Pricing for the Nature of AI
Most traditional pricing models are based on the idea that usage grows in a steady, linear fashion. That assumption works for many SaaS products, but it falls apart when applied to AI. From training large language models to running inference pipelines, AI workloads are inherently unpredictable. They scale up fast, vary between teams, and shift based on data complexity and compute requirements.
In this kind of environment, rigid tiers become a liability. Go over your usage threshold, and you’re hit with unexpected charges. Over-provision to avoid that risk, and you’re wasting budget. Either way, you're forced to make pricing decisions that are disconnected from your actual needs.
This is where AI dynamic pricing offers a better path. Instead of forcing customers into fixed categories, it adapts to changing patterns in real time. This kind of dynamic pricing strategy gives organizations the flexibility to scale without fear, and the clarity to predict costs with confidence.
More importantly, it aligns pricing with how AI is truly used. Whether you’re experimenting with a new use case or ramping up production workloads, flexible AI pricing makes it possible to match your spend with your usage. No more, no less. It’s a smarter approach to pricing that prioritizes transparency, trust, and long-term value creation.
And it’s becoming more relevant by the day. Nearly 70% of companies that have built AI products are already monetizing them or testing monetization, up from 63% in 2023. The majority are doing so through subscription or hybrid pricing models with a subscription component. While less than 10% are currently using result- or output-driven models, we expect this to grow.
As vendors become better at proving direct outcomes from AI, pricing will likely evolve to reflect those results, aligning value capture more closely with customer success and strengthening net revenue retention in the process.
What Makes a Pricing Model “Dynamic”?
A dynamic AI pricing model is built around one core principle: adapt to usage in real time. Instead of locking customers into fixed tiers, it offers the flexibility to scale up or down based on actual demand, without hidden fees or painful overages.
This isn’t just a more forgiving billing model. It’s a pricing strategy designed to reflect how AI is used in the real world. Think burst usage during model training, inconsistent demand across customer segments, or compute-intensive experiments that don’t follow a predictable schedule.
Leading companies are already embracing dynamic pricing models like:
- Burst pricing, which allows for short-term spikes by charging a marginal per-unit rate only when usage exceeds an agreed threshold.
- Spot consumption, where customers tap into unused capacity at a discount, perfect for non-critical or interruptible workloads.
- Credit-based models, where customers buy flexible units (credits, tokens, or AI units) that can be used across different services as needs shift.
These models give customers control. They can monitor usage, plan budgets, and make smarter pricing decisions. And for vendors, dynamic models create better alignment between value delivered and revenue captured.
It’s worth noting: this isn’t about gimmicks or discounts. It’s about using AI algorithms and machine learning to track patterns, segment usage, and adjust pricing accordingly. Done right, it helps companies offer competitive prices, optimize margins, and build long-term customer relationships rooted in trust.
Real-World Examples of Dynamic Pricing in Action
The shift to dynamic B2B pricing is already happening. Leading tech companies are rethinking how they bill for AI and data infrastructure, moving away from one-size-fits-all models in favor of more responsive, customer-centric approaches.
Google Cloud Storage, for example, introduced a unique forgiveness policy that helps reduce customer anxiety around unpredictable demand. Customers commit to a fixed spend over 12 months, but if usage exceeds that by up to 30%, the overage is waived, as long as the customer commits to the higher level going forward. This approach rewards growth without penalizing success and supports a more stable, scalable pricing relationship.
Sumo Logic takes a different route with its credit-based pricing model. Customers purchase Cloud Flex Credits that can be applied across multiple services like logging, storage, and metrics, based on current needs. This model supports adjusting prices based on shifting demand across departments or projects, and helps avoid lock-in to specific SKUs. It’s especially effective in AI environments where teams need to pivot quickly without blowing the budget.
On the flip side, Informatica shows what happens when dynamic pricing lacks transparency. While it also uses credits, the pricing logic behind them varies widely: some services consume 0.19 credits per compute unit, while others may burn through 13 credits per million API calls. Without clear communication, these inconsistencies create confusion and make it harder for customers to forecast spend. According to Gartner, such unclear pricing mechanics can damage trust and limit adoption.
The takeaway? Implementing dynamic pricing works best when it’s predictable, flexible, and clearly communicated. Whether it’s forgiving overages, letting customers shift spend between services, or aligning with actual usage patterns, the goal is always the same: reduce friction and support smarter growth.
Building an AI Pricing Strategy That Works
A solid AI pricing strategy is about more than just billing mechanics, it’s about aligning value, usage, and customer expectations. As AI adoption grows, pricing needs to reflect the diversity of use cases and the volatility of demand. That means moving away from rigid structures toward something modular, intelligent, and customer-first.
Smart vendors are now adjusting prices in real time, offering multiple entry points that suit different customer segments. An enterprise may start with a flat-rate subscription but need burst capacity when launching a new AI model. A mid-market team might prefer usage-based pricing with built-in thresholds, alerts, and the ability to monitor spend. Both benefit from transparency and flexibility.
Visibility is a key piece of this puzzle. When users can track consumption, model future costs, and receive real-time notifications, they’re empowered to make better decisions and far less likely to be surprised by their invoice. This also cuts down on support costs and positions the vendor as a strategic partner, not a gatekeeper.
Trust also plays a critical role. That’s why the best dynamic pricing models bake in customer-friendly policies:
- Rollover options for unused credits
- Flexible upgrade paths mid-contract
- Fairness clauses that prevent punitive overage fees
These features aren’t just nice-to-haves, they’re actual growth drivers. They reduce churn, improve satisfaction, and increase expansion potential. When pricing is designed to maximize revenue and help customers succeed, everyone wins.
It’s also worth watching the trend: Gartner predicts that credit-based pricing will account for over 25% of net new spend with the top ten enterprise software vendors by 2027. That’s a clear signal that the future belongs to those who rethink their approach to pricing and execute it well.
From Billing Model to Product Strategy
Pricing is more than just a back-office task, it’s core product strategy. As AI reshapes everything from infrastructure to user workflows, vendors must ensure their monetization models evolve in parallel. Rigid tiers and hidden fees are no longer acceptable. Customers expect pricing that is clear, responsive, and built for how they actually use the product.
And the stakes are high. In a recent survey, 60% of companies said pricing complexity was one of the biggest blockers to scaling AI. That’s not just a billing issue, but a growth bottleneck.
This is where AI dynamic pricing becomes a competitive edge. When you build pricing that flexes with demand, reflects real-world usage, and responds to shifting workloads, you’re improving the product experience.
More importantly, you’re using the same intelligence that powers your AI to inform how you charge for it. With the help of machine learning algorithms, vendors can offer optimal pricing based on usage patterns, price sensitivity, and customer behavior. Whether you’re serving online retailers, mid-sized enterprises, or global tech platforms, the ability to adjust their pricing based on demand and customer value is fast becoming a must-have.
At Vayu, we see pricing as a living part of the product. That means embedding flexibility, transparency, and intelligence into every interaction, from the first quote to the renewal conversation. In a world defined by constant change, the smartest pricing model is one that’s built to adapt.
FAQ
1. What is dynamic AI pricing, and how is it different from traditional SaaS pricing?
Dynamic AI pricing adjusts in real time based on actual usage, unlike traditional SaaS pricing which relies on fixed tiers. This flexibility ensures customers only pay for what they use, making it ideal for unpredictable AI workloads.
2. What are the benefits of dynamic pricing for AI-driven organizations?
It reduces surprise overages, improves cost predictability, and aligns spend with value delivered. Dynamic pricing models also build trust by offering transparency, flexible usage options, and fairness policies that reflect real-world demand.
3. How can vendors implement dynamic AI pricing effectively?
Success depends on clear communication, smart usage tracking, and customer-friendly policies like rollover credits and usage alerts. Leveraging machine learning to segment usage and optimize price points can further align pricing with customer value.